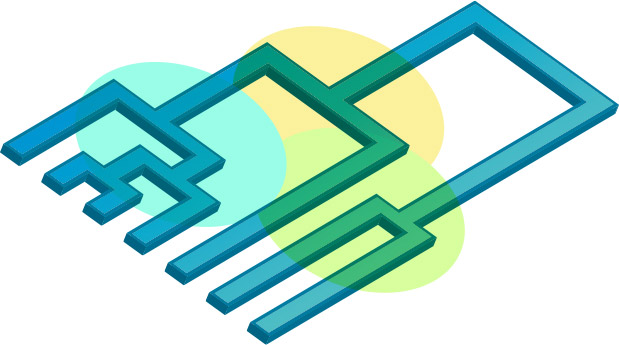
2/CLU
CLUSTER ANALYSIS
THE PRODUCT
2/CLU is our tool for quick, easy and stable segmentation of a large number of objects into homogeneous clusters
FEATURES IN BRIEF
- Supports all common hierarchical and partitioning procedures
- Combination of both approaches, to determine a stable starting partition and for the stepwise optimisation of this solution (two-stage clustering)
- Also allows the grouping of cases with missing values
- Delivers quality criteria as the basis for decisions to determine the “right“ number of clusters
- Offers extensive options for the individual design of graphics to clearly and concisely display the identified clusters
OBJECTIVE
The goal of a cluster analysis is to break down a heterogeneous group of objects into homogenous subgroups.
The groups thus found would not be identifiable through conventional group formation, for instance by age group, gender, income, etc. They contain objects which are as similar as possible in terms of the attributes employed. One example of this would be persons who share the same opinion on a particular topic. Thus, it becomes possible to assist marketing departments in addressing target groups in the most appropriate manner.
PREREQUISITES
The characteristics by which persons are to be classified must be specified in advance (active variables). Typically, statement batteries are used for this purpose. These may cover e.g. the opinions held by respondents on particular subjects.
The scale used makes no difference to the calculation, but all active variables should use an identical scale. If questions with differing scales are being used, these must first be standardized to the same scale.
As a standard rule of thumb, every cluster should include at least 50 persons. We recommend a sample size of at least 300 persons.
When specifying active variables, it should be kept in mind that these should not exhibit an overly high level of correlation with one another. The redundant information would have an excessively high impact on the formation of groups. However in real life this can never be completely eliminated.
To avoid high levels of correlation, it is sometimes advisable to include a Factor Analysis.